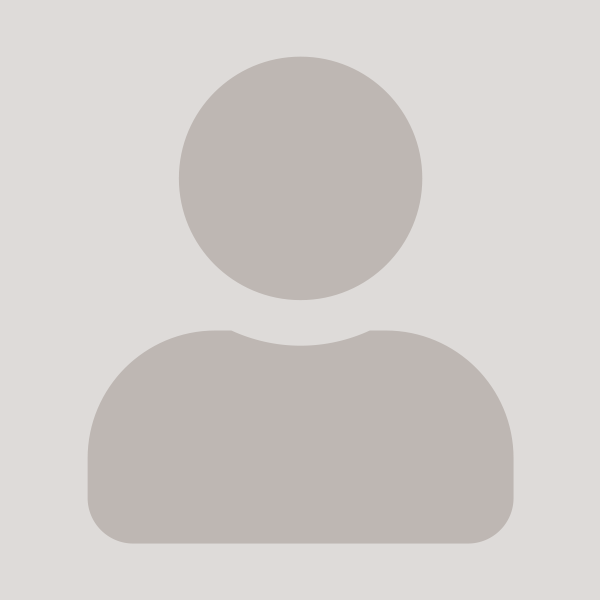
Carsten Peterson
Expert
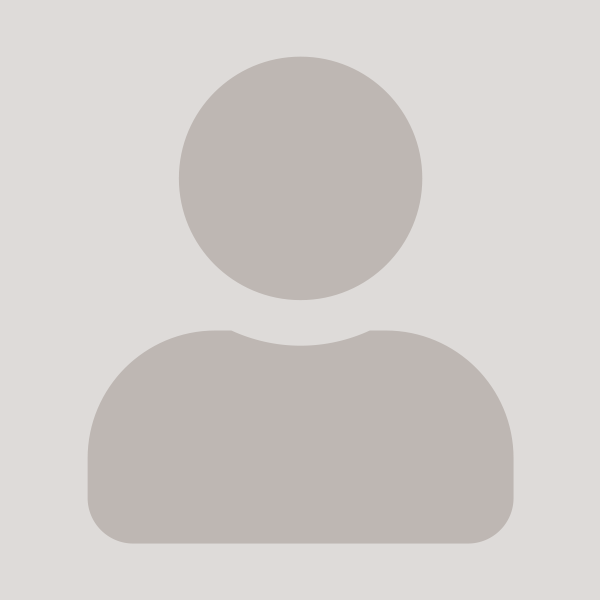
A New Method for Mapping Optimization Problems onto Neural Networks
Author
Summary, in English
A novel modified method for obtaining approximate solutions to difficult optimization problems within the neural network paradigm is presented. We consider the graph partition and the travelling salesman problems. The key new ingredient is a reduction of solution space by one dimension by using graded neurons, thereby avoiding the destructive redundancy that has plagued these problems when using straightforward neural network techniques. This approach maps the problems onto Potts glass rather than spin glass theories. A systematic prescription is given for estimating the phase transition temperatures in advance, which facilitates the choice of optimal parameters. This analysis, which is performed for both serial and synchronous updating of the mean field theory equations, makes it possible to consistently avoid chaotic behavior.
When exploring this new technique numerically we find the results very encouraging; the quality of the solutions are in parity with those obtained by using optimally tuned simulated annealing heuristics. Our numerical study, which for TSP extends to 200-city problems, exhibits an impressive level of parameter insensitivity.
When exploring this new technique numerically we find the results very encouraging; the quality of the solutions are in parity with those obtained by using optimally tuned simulated annealing heuristics. Our numerical study, which for TSP extends to 200-city problems, exhibits an impressive level of parameter insensitivity.
Department/s
- Computational Biology and Biological Physics - Has been reorganised
Publishing year
1989
Language
English
Pages
3-22
Publication/Series
International Journal of Neural Systems
Volume
1
Issue
1
Document type
Journal article
Publisher
World Scientific Publishing
Topic
- Computational Mathematics
- Other Physics Topics
Status
Published
ISBN/ISSN/Other
- ISSN: 0129-0657