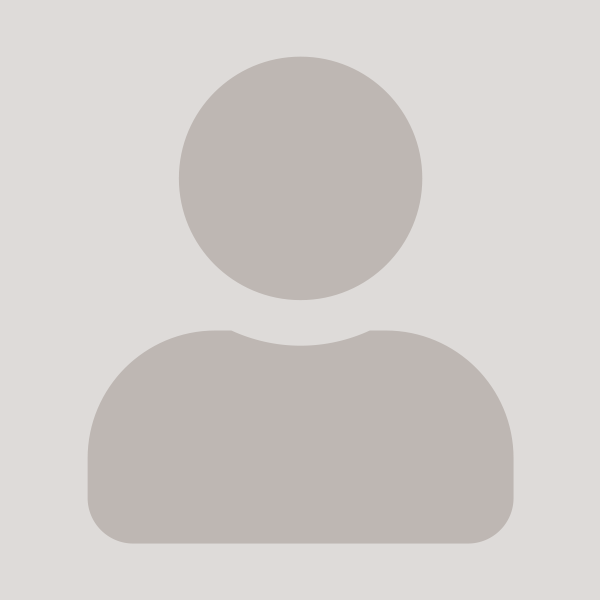
Carsten Peterson
Expert
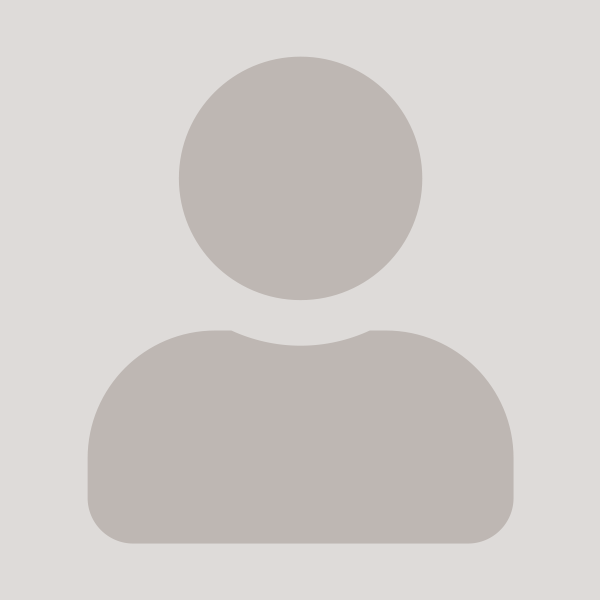
Solving optimization problems with mean field methods
Author
Summary, in English
A brief review is given for the use of feed-back artificial neural networks (ANN) to obtain good approximate solutions to combinatorial optimization problems. The key element is the mean field approximation (MFT), which differs from conventional methods and "feels" its ways towards good solutions rather than fully or partly exploring different possible solutions. The methodology, which is illustrated for the graphs bisection and knapsack problems, is easily generalized to Potts systems. The latter is related to the deformable templates method, which is illustrated with the track finding problem. The mean field approximation is based on a variational principle, which also turns out to be very profitable when computing correlations in polymers.
Department/s
- Department of Astronomy and Theoretical Physics - Has been reorganised
Publishing year
1993-11-15
Language
English
Pages
570-580
Publication/Series
Physica A: Statistical Mechanics and its Applications
Volume
200
Issue
1-4
Document type
Journal article
Publisher
Elsevier
Topic
- Computational Mathematics
Status
Published
ISBN/ISSN/Other
- ISSN: 0378-4371