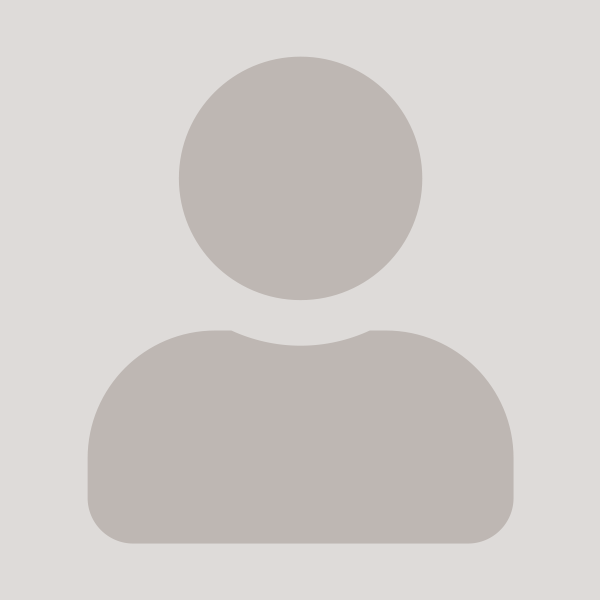
Bo Söderberg
Teaching staff
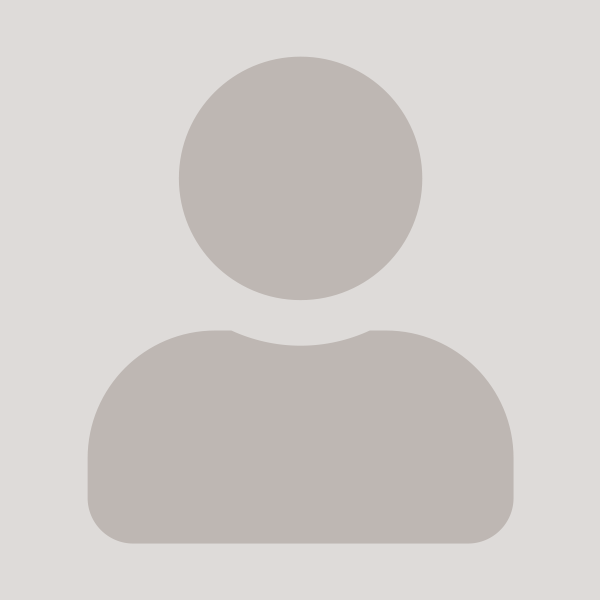
An information-based neural approach to constraint satisfaction
Author
Summary, in English
A novel artificial neural network approach to constraint satisfaction problems is presented. Based on information-theoretical considerations, it differs from a conventional mean-field approach in the form of the resulting free energy. The method, implemented as an annealing algorithm, is numerically explored on a testbed of K-SAT problems. The performance shows a dramatic improvement over that of a conventional mean-field approach and is comparable to that of a state-of-the-art dedicated heuristic (GSAT+walk). The real strength of the method, however, lies in its generality. With minor modifications, it is applicable to arbitrary types of discrete constraint satisfaction problems.
Department/s
- Computational Biology and Biological Physics - Has been reorganised
Publishing year
2001-08
Language
English
Pages
1827-1838
Publication/Series
Neural Computation
Volume
13
Issue
8
Document type
Journal article
Publisher
MIT Press
Status
Published
ISBN/ISSN/Other
- ISSN: 0899-7667