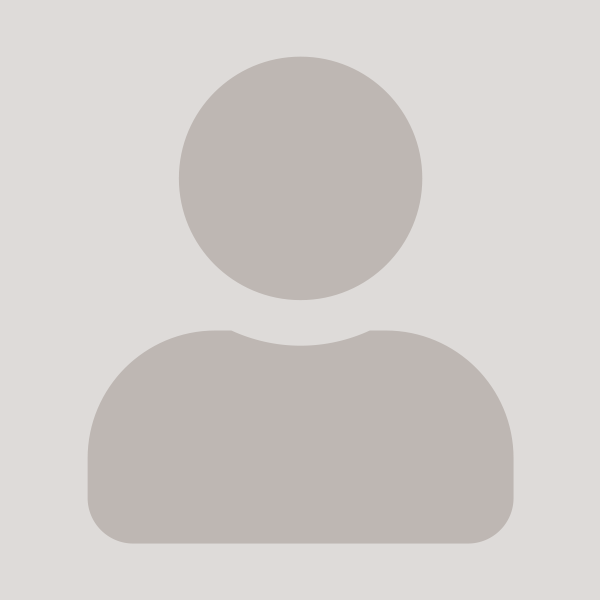
Bo Söderberg
Teaching staff
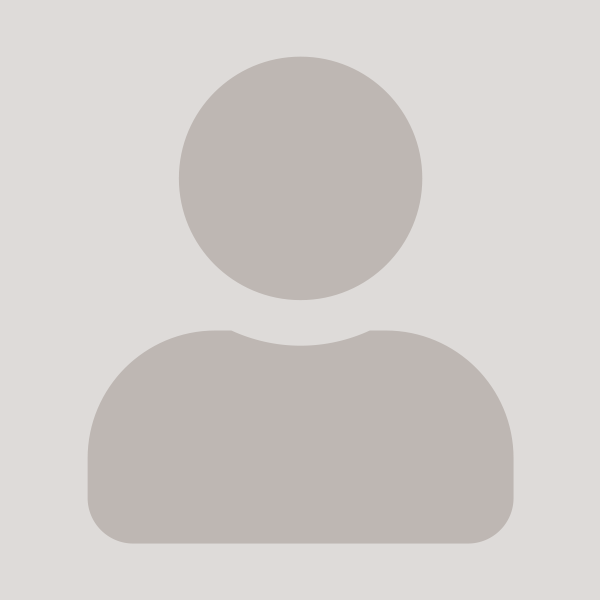
An information-based neural approach to generic constraint satisfaction
Author
Summary, in English
A novel artificial neural network heuristic (INN) for general constraint satisfaction problems is presented. extending a recently suggested method restricted to boolean variables. In contrast to conventional ANN methods, it employs a particular type of non-polynomial cost function, based on the information balance between variables and constraints in a mean-field setting. Implemented as an annealing algorithm, the method is numerically explored on a testbed of Graph Coloring problems. The performance is comparable to that of dedicated heuristics, and clearly superior to that of conventional mean-field annealing.
Department/s
- Computational Biology and Biological Physics - Has been reorganised
Publishing year
2002
Language
English
Pages
1-17
Publication/Series
Artificial Intelligence
Volume
142
Issue
1
Document type
Journal article
Publisher
Elsevier
Topic
- Biophysics
Keywords
- constraint satisfaction
- connectionist
- artificial
- neural network
- heuristic information
- mean-field annealing
- graph coloring
Status
Published
ISBN/ISSN/Other
- ISSN: 1872-7921